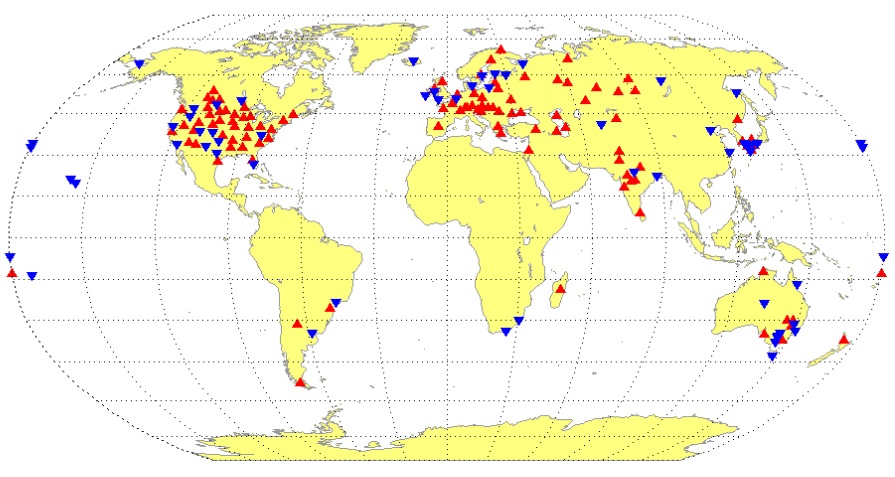
Koutsoyiannis and Steirou analysed 163 time series. 2/3 of the stations are adjusted upwards leading to a warm bias in the global surface land temperature.
My last post (in Dutch) on the new paper of Dick McNider reminded me of a very interesting presentation I attended at the last EGU meeting in Vienna. The presentation was given by Eva Steirou, a scientist in the group of Demetris Koutsoyiannis.
The popular summary of the presentation is that the rise in global land temperatures is probably smaller than the generally accepted 0,7-0,8 °C. Steirou and Koutsoyiannis claim that the adjustments that are applied to the raw monthly GHCN data make the trend larger.
They are not the first of course who claim this is the case. There are many examples on Climate Audit and Watts Up With That? showing a rising trend for individual stations due to adjustments. And just today a High Court case in New Zealand has started dealing with the accusation that most of the trend there is based on adjustments only.
However there are also interesting analyses done by amongst others Zeke Hausfather and Steven Mosher, that show very little difference between GHCN raw and adjusted data on a global scale.
Now for their analysis Koutsoyiannis and Steirou used only a subset of GHCN. They selected time series with 100 years of data and few missing months (see page 9 of their presentation). They end up using 163 series, visible on the map at the top of this post. Very few stations in Africa (3) and South America (5) pass the selection criteria.
The warming trend based on the raw data (I suppose in this case just a simple average of the 163 series) is 0,42 °C versus 0,76 °C for the adjusted data. Their analysis shows that 2/3 of the stations are adjusted upwards, where the expected proportion would be 1/2.
Next the researchers used synthetic time series to test the Standard Normal Homogeneity Test (SNHT) used by GHCN. They showed that SNHT was able to detect an artificial shift in independent normally ditributed data. However when the synthetic data contained long-term persistence (as is the case with real world temperature series) the program identified shifts in the data that weren’t there. As a result SNHT generated a trend that wasn’t there. The researchers conclude that SNHT doesn’t seem to be the best choice when dealing with time series showing long term persistence.
Marcel, Tamino would like to know: Where is the skepticism?
“Their analysis shows that 2/3 of the stations are adjusted upwards, where the expected proportion would be 1/2.” If the inhomogeneities would only due to relocations, one would expect the proportion to be 1/2. However, we know that the climate record contains biases. In the beginning of the observations there were, for example, still problems with the radiation protection of the thermometers. These problems were small relative to the day to day variations of the weather, but to study climate change they need to be removed to obtain an honest appraisal of the change in the climate in stead of… Lees verder »